|
5 | 5 | Paddle Graph Learning (PGL) is an efficient and flexible graph learning framework based on [PaddlePaddle](https://github.com/PaddlePaddle/Paddle).
|
6 | 6 |
|
7 | 7 |
|
8 |
| - |
| 8 | +<img src="https://github.com/PaddlePaddle/PGL/blob/master/docs/source/_static/framework_of_pgl.png" alt="The Framework of Paddle Graph Learning (PGL)" width="800"> |
9 | 9 |
|
10 | 10 |
|
11 | 11 | We provide python interfaces for storing/reading/querying graph structured data and two fundamental computational interfaces, which are walk based paradigm and message-passing based paradigm as shown in the above framework of PGL, for building cutting-edge graph learning algorithms. Combined with the PaddlePaddle deep learning framework, we are able to support both graph representation learning models and graph neural networks, and thus our framework has a wide range of graph-based applications.
|
12 | 12 |
|
13 | 13 |
|
14 | 14 | ## Highlight: Efficient and Flexible Message Passing Paradigm
|
15 | 15 |
|
16 |
| -One of the most important benefits of graph neural networks compared to other models is the ability to use node-to-node connectivity information, but coding the communication between nodes is very cumbersome. At PGL we adopt **Message Passing Paradigm** similar to [DGL](https://github.com/dmlc/dgl) to help to build a customize graph neural network easily. Users only need to write ```send``` and ```recv``` functions to easily implement a simple GCN. As shown in the following figure, for the first step the send function is defined on the edges of the graph, and the user can customize the send function $\phi^e$ to send the message from the source to the target node. For the second step, the recv function $\phi^v$ is responsible for aggregating $\oplus$ messages together from different sources. |
| 16 | +One of the most important benefits of graph neural networks compared to other models is the ability to use node-to-node connectivity information, but coding the communication between nodes is very cumbersome. At PGL we adopt **Message Passing Paradigm** similar to [DGL](https://github.com/dmlc/dgl) to help to build a customize graph neural network easily. Users only need to write ```send``` and ```recv``` functions to easily implement a simple GCN. As shown in the following figure, for the first step the send function is defined on the edges of the graph, and the user can customize the send function 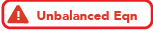 to send the message from the source to the target node. For the second step, the recv function 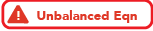 is responsible for aggregating 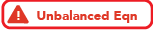 messages together from different sources. |
17 | 17 |
|
18 |
| - |
19 | 18 |
|
20 | 19 |
|
21 |
| -As shown in the left of the following figure, to adapt general user-defined message aggregate functions, DGL uses the degree bucketing method to combine nodes with the same degree into a batch and then apply an aggregate function $\oplus$ on each batch serially. For our PGL UDF aggregate function, we organize the message as a [LodTensor](http://www.paddlepaddle.org/documentation/docs/en/1.4/user_guides/howto/basic_concept/lod_tensor_en.html) in [PaddlePaddle](https://github.com/PaddlePaddle/Paddle) taking the message as variable length sequences. And we **utilize the features of LodTensor in Paddle to obtain fast parallel aggregation**. |
| 20 | +<img src="https://github.com/PaddlePaddle/PGL/blob/master/docs/source/_static/message_passing_paradigm.png" alt="The basic idea of message passing paradigm" width="800"> |
22 | 21 |
|
| 22 | +As shown in the left of the following figure, to adapt general user-defined message aggregate functions, DGL uses the degree bucketing method to combine nodes with the same degree into a batch and then apply an aggregate function 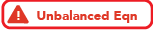 on each batch serially. For our PGL UDF aggregate function, we organize the message as a [LodTensor](http://www.paddlepaddle.org/documentation/docs/en/1.4/user_guides/howto/basic_concept/lod_tensor_en.html) in [PaddlePaddle](https://github.com/PaddlePaddle/Paddle) taking the message as variable length sequences. And we **utilize the features of LodTensor in Paddle to obtain fast parallel aggregation**. |
23 | 23 |
|
24 |
| - |
25 | 24 |
|
| 25 | +<img src="https://github.com/PaddlePaddle/PGL/blob/master/docs/source/_static/parallel_degree_bucketing.png" alt="The parallel degree bucketing of PGL" width="800"> |
26 | 26 |
|
27 | 27 |
|
28 | 28 | Users only need to call the ```sequence_ops``` functions provided by Paddle to easily implement efficient message aggregation. For examples, using ```sequence_pool``` to sum the neighbor message.
|
@@ -54,7 +54,8 @@ We test all the GNN algorithms with Tesla V100-SXM2-16G running for 200 epochs t
|
54 | 54 | PGL requires:
|
55 | 55 |
|
56 | 56 | * paddle >= 1.5
|
57 |
| -* networkx |
| 57 | +* networkx |
| 58 | +* cython |
58 | 59 |
|
59 | 60 |
|
60 | 61 | PGL supports both Python 2 & 3
|
|
0 commit comments